Understanding and managing customer churn is crucial for any SaaS company looking to sustain growth and ensure customer retention.
Predicting and preventing churn helps in maintaining a loyal customer base and reducing the costs associated with acquiring new customers.
This article will delve into the importance of customer churn prediction and offer actionable strategies to improve retention.
You'll learn:
- Types of churn and their implications
- How to create and use churn prediction models
- Steps to effectively predict and prevent churn
- The role of data integration and management in churn prediction
- Common reasons for customer churn
- Strategies to retain existing customers
What is churn prediction?
Customer churn prediction identifies which customers are likely to stop using a service by analyzing historical data, customer behavior, and predictive models. This proactive approach helps businesses address potential issues and retain high risk customers.
For SaaS companies, churn prediction is vital due to the subscription-based revenue model. It helps allocate resources effectively, focusing on retention rather than constant customer acquisition, reducing costs and enhancing customer lifetime value.
Common techniques include logistic regression, decision trees, neural networks, and ensemble methods. These models analyze data points like usage patterns, transaction history, and engagement metrics to identify at-risk customers.
Accurate churn prediction improves customer satisfaction, loyalty, and revenue growth.
By predicting churn, you can implement targeted retention strategies and foster long-term relationships.
Types of churn

Understanding the different types of churn is essential for developing effective retention strategies.
Churn can be broadly categorized as follows:
Voluntary Churn: This occurs when customers choose to leave due to dissatisfaction with the product, better alternatives, or changes in their needs. It's often driven by factors such as poor customer experience, high prices, or unmet expectations.
Involuntary Churn: This happens when customers are lost due to reasons beyond their control, such as payment failures or expired credit cards. These customers often leave unintentionally and might not be aware their subscription has ended.
Active Churn: Active churn involves customers explicitly canceling their subscriptions. This type of churn is usually deliberate and can be due to a clear dissatisfaction or a decision to switch to a competitor.
Passive Churn: This occurs when customers churn by stopping using the service without formally canceling their subscription. These customers may have lost interest or found an alternative, but they don’t take the step to cancel, often leading to unnoticed revenue loss until their subscription naturally expires.
Revenue Churn: This type of churn measures the loss of revenue from existing customers. It includes customers who downgrade their plans or reduce their usage, thereby decreasing the overall revenue.
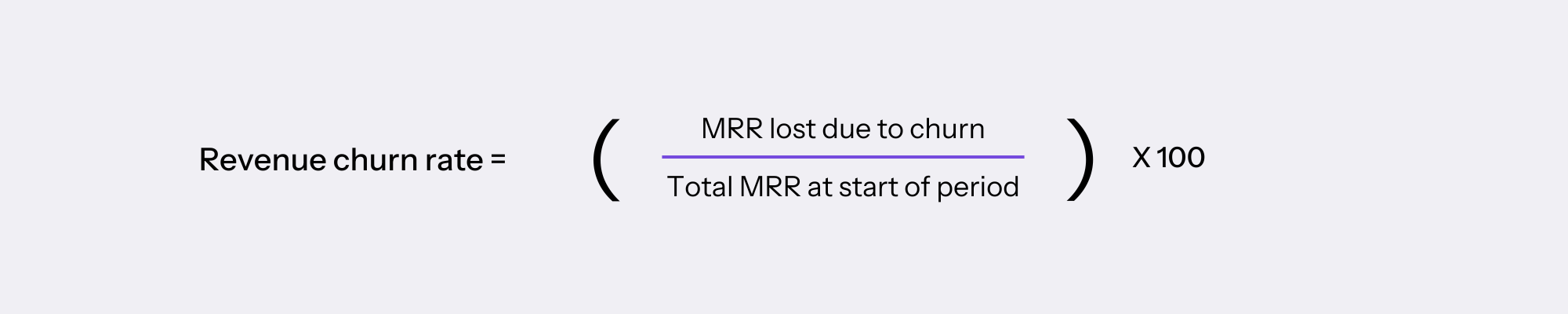
Customer Churn: Customer churn measures the number of customers who stop using the service within a given period. It's a direct indicator of customer loss and helps businesses understand the scale of their retention challenges.

How churn affects businesses
Customer churn impacts several critical areas of a business, each influencing its overall health and growth.
- Revenue: High churn rates directly reduce recurring revenue. Every lost customer translates to a loss in potential earnings, impacting the financial stability of a business.
- Product development: A high churn rate indicates product issues or unmet customer needs. Insights from churn analysis can guide improvements and innovations, ensuring the product evolves to meet customer expectations.
- Customer acquisition costs: Acquiring new customers is significantly more expensive than retaining existing ones. High churn necessitates increased spending on marketing and sales efforts to replace lost customers, straining budgets.
- Brand reputation: A high churn rate can damage a company’s reputation. Dissatisfied customers may share negative feedback, influencing potential customers' perceptions and making it harder to attract new business.
- Employee morale: Consistent churn can demoralize employees, particularly those in customer-facing roles. Seeing customers leave despite their efforts can lead to frustration and decreased motivation.
- Market insights: Churn data provides valuable insights into market trends and customer preferences. Understanding why customers leave can help businesses adapt strategies to better align with market demands.
- Overall business growth: Churn hampers long-term growth by reducing the customer base and revenue streams. A focus on retention is essential for sustainable business expansion and achieving growth targets.
4 steps to predict churn
1. Comprehensive customer segmentation
Churn prediction relies heavily on analyzing your company’s historical customer data. Start by exporting all relevant data types that could influence a customer’s likelihood to churn.
These data points can include demographics, behavioral data, usage rates, support ticket submissions, revenue information, subscription dates, and contract terms.
For instance, understanding whether a user is an individual or a company representative, their frequency of product use, the number of support tickets they submit, and their subscription duration can provide critical insights.
Segment users based on their churn risk within these categories:
- High-engagement customers with frequent upgrades (low risk of churn)
- Regularly communicating customers (low risk of churn)
- Users with no or repetitive support tickets (risk of churn)
- Customers with declining product use (high risk of churn)
- New users who didn’t complete onboarding (high risk of churn)
2. Manual data analysis or predictive services
After gathering and segmenting your data, decide whether to continue with manual analysis or use a predictive service.
In-house solutions allow customization based on your data and the preferred methodologies of your data science team, such as a machine learning model for complex data relationships or survival analysis to predict customer churn over specific time periods.
If you don't have a data scientist or the required resources to build your own machine learning model, you can use tools such as Amplitude, UserPilot, or Paddle, which focus on forecasting and preventing churn.
3. Analyzing data to identify churn trends
Once you’ve segmented your customers and analyzed their data, identify the patterns and correlations that indicate churn risk.
For example, a high number of customers may churn after sign-up due to confusing pricing tiers, or long-time customers might churn because of a lack of communication, leading to unnoticed credit card expirations.
Churn prediction models can uncover these patterns, allowing proactive measures to address and prevent customer churn. Real-time alerts can also help detect immediate churn risks, such as missed payments, which signal waning customer interest.
4. Customer retention strategies
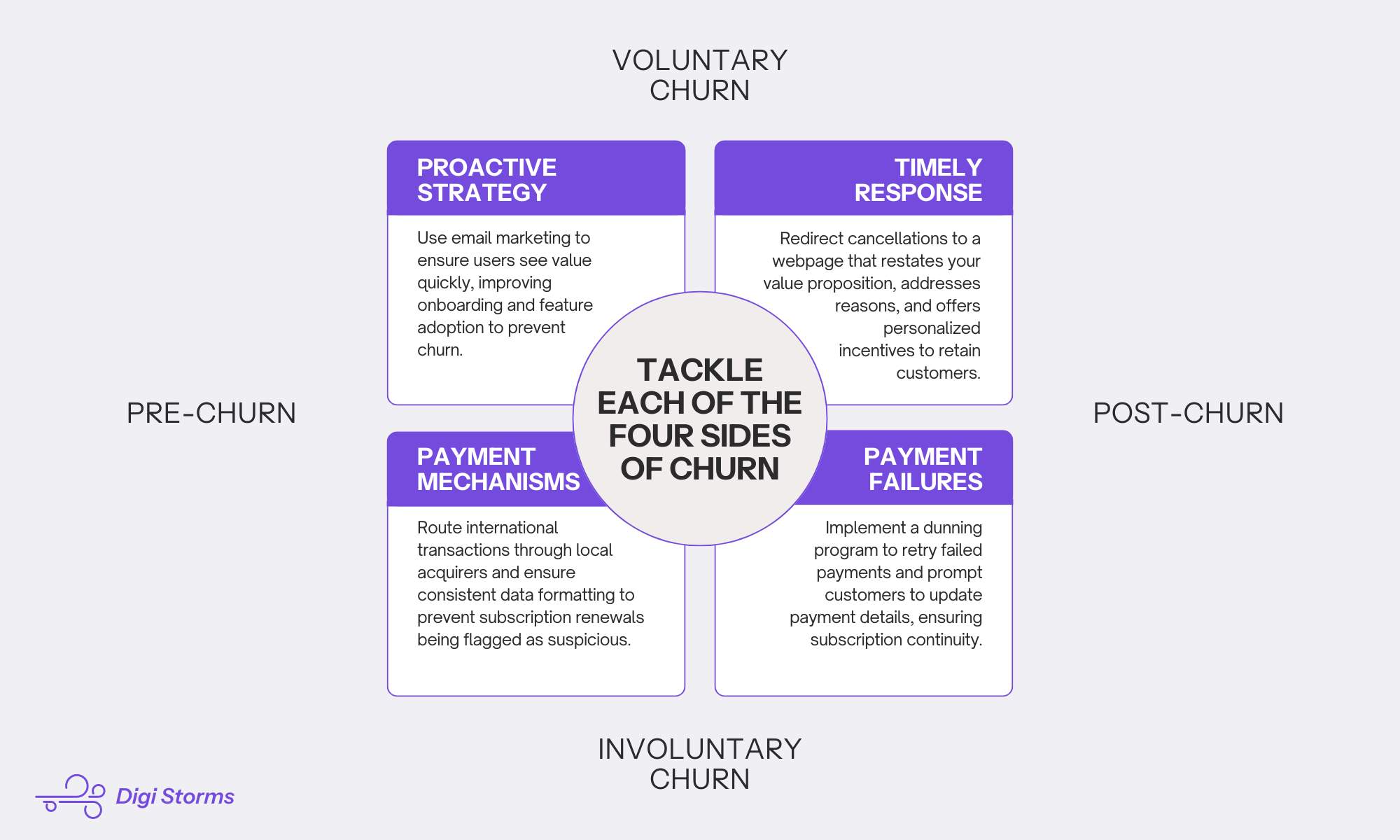
With a clear understanding of churn trends and of which customer segments are at risk, develop strategies to address their specific issues:
- Post-sign-up churn: Ensure your pricing page is clear and help customers during onboarding to address immediate concerns.
- Long-time customer churn: Offer targeted incentives like discounts, special upgrades, or loyalty bonuses to keep veteran customers engaged. Maintain regular communication, especially before contract renewals.
- Churn after product updates: Inform customers about updates well in advance and provide resources to help them navigate new features or improvements.
By following these steps, you should be able to better predict and prevent customer churn, ultimately enhancing customer retention and driving long-term growth.
Now let's get a bit more technical and see how to build churn prediction models.
How to prevent churn and keep existing customers
1. Define your churn prediction objectives
To build an effective customer churn prediction model, start by identifying your goals. These typically include reducing customer attrition by pinpointing which customers are at risk of churning, understanding the underlying reasons for their potential departure, and designing interventions to improve retention.
Clearly defined objectives ensure that your model targets the most relevant aspects of user behavior.
2. Prepare your data
💡 Collecting and organizing relevant customer data is crucial for building a robust churn prediction model. This data can come from CRMs, customer analytics software, or direct customer feedback, encompassing various touchpoints in the customer journey.
Ensuring the data is accurate and comprehensive is key. Tools like Amplitude Audiences can help streamline this process by providing detailed guides on data collection and preparation.
3. Feature engineering and selection
Feature engineering involves identifying and categorizing the attributes that influence churn. Key features include:
- Customer features: Demographic information such as age, income, and education level.
- Support features: Interaction data with customer support, including the number of emails, resolution times, and satisfaction ratings.
- Usage features: How customers use your product, like average call duration or login frequency.
- Contextual features: Information about the customer's environment, such as device OS or purchase history.
- Behavioral features: Specific actions within the product, such as sharing content or app uninstallations.
After identifying these features, standardize and select only the relevant ones for churn analysis.
4. Build your Machine learning model

Machine learning models, especially those focused on binary classification, are commonly used in churn prediction. These models categorize features into true or false outcomes based on their impact on churn.
Decision trees and random forests are popular choices. Decision trees provide various scenarios based on available features, while random forests use multiple decision trees to enhance churn prediction accuracy through majority voting.
We'll see how to build four different models in the next part.
5. Monitore and refine your model
Once deployed, continuously monitor your model’s performance. Use predictive tools to test and track its accuracy, adjusting features as necessary. Effective monitoring ensures accurate predictions over time and adapts to changing customer behaviors.
If successful, the model can be integrated into existing applications or form the basis of new retention-focused products.
Choosing the right customer churn prediction model
Creating effective models to predict churn involves several techniques, each suited to different types of data and business needs. Here are some of the most common machine learning algorithms:
Logistic regression
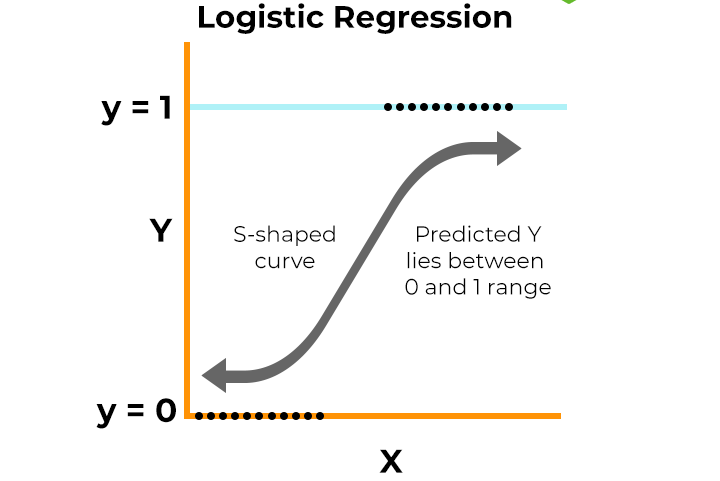
Logistic regression is a statistical technique used to predict binary outcomes, such as whether a customer will leave or stay. This model uses predictors like demographics, purchase history, and customer interactions to estimate the probability of churn.
By analyzing historical data, logistic regression calculates the odds of churn for each variable, highlighting the most predictive factors. For instance, a high number of service complaints may indicate a higher likelihood of churn.
This method is well-suited for businesses with extensive user data and clear binary outcomes, such as telecoms, finance, and subscription based companies. Logistic regression is easy to implement and provides actionable insights, though it may struggle with complex data relationships.
Decision trees

Decision trees are popular for modeling customer churn prediction. They break down decisions into a series of binary choices, creating a tree-like structure based on customer attributes and behaviors, such as usage patterns and service levels.
Each node in the tree represents a test for an attribute, and each branch represents the outcome of that test, leading to a final decision (churn or no churn). Decision trees are intuitive and user-friendly, making them easy to understand and communicate. They work well for businesses with diverse customer bases where various factors influence churn.
However, the decision tree model can overfit training data, capturing noise rather than underlying patterns, and may struggle with tasks requiring linear relationships.
Neural networks

Neural networks, a type of machine learning, capture complex, non-linear relationships within data. They consist of layers of interconnected nodes that process input data hierarchically.
In churn prediction, a neural network processes user data through its layers to produce a probability of churn. Neural networks learn from data by adjusting the weights of connections based on observed patterns, improving prediction accuracy over time. They are beneficial for industries with vast customer interaction data, such as telecoms, streaming services, and online retail.
While neural networks can model complex relationships, they are challenging to interpret, require substantial data preparation, and are computationally intensive, necessitating more resources and expertise.
Ensemble method

Ensemble methods combine multiple predictive machine learning algorithms to improve accuracy. These methods train different models on the same data and aggregate their predictions using techniques like bagging, boosting, or stacking.
In churn prediction, ensemble methods might combine decision trees, neural networks, and logistic regression models, each predicting churn independently. The final prediction is derived from a combination of these models, enhancing accuracy. Ensemble methods are useful in scenarios where no single model provides satisfactory predictions or where data is intricate and diverse.
They improve reliability and stability by reducing overfitting, though they require more resources and time to train and deploy and can be difficult to interpret.
Data management in predicting customer churn
The importance of quality data preparation
⚠️ The foundation of any effective customer churn prediction model is the quality of the data it uses. Collecting comprehensive and accurate data is crucial. This involves gathering detailed information about customers and ensuring it is relevant and up to date.
Equally important is integrating data from various sources into a single, coherent database, which is essential for creating a holistic view of your customers.
Accurate and well-integrated data help develop a reliable churn prediction model, leading to better decision-making and retention strategies.
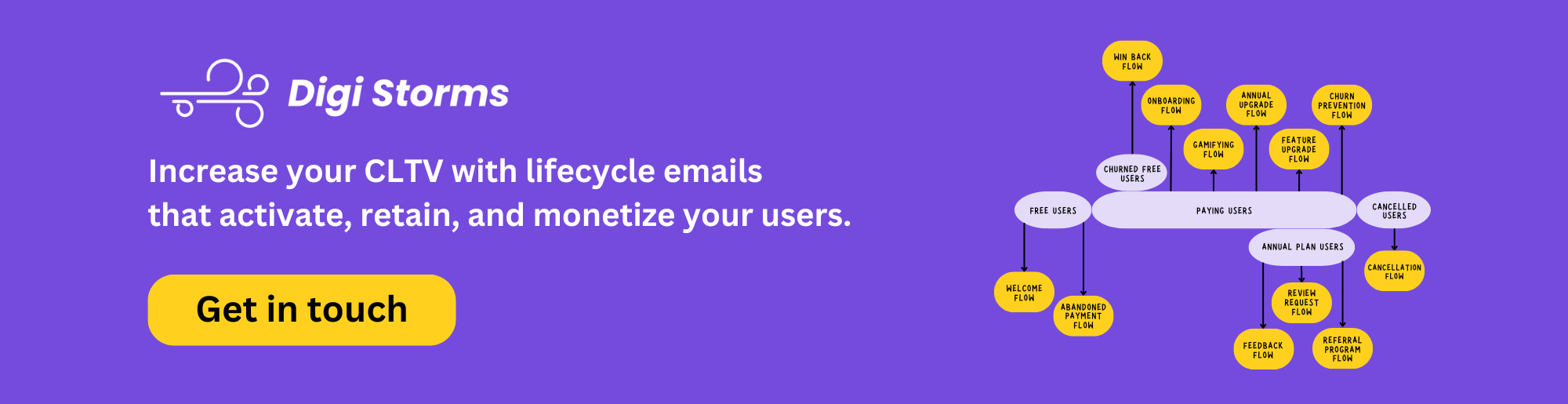
Exploring various data sources
A diverse range of data sources can inform churn prediction. Key sources include:
- Transactional data: This includes purchase history, payment methods, and product or service usage patterns. Transactional data offers direct insights into user behavior and preferences, which are vital to predict customer churn.
- Customer interactions: Records of customer service interactions, feedback, and complaints are invaluable. They provide a window into customer satisfaction and potential pain points, helping identify factors that may lead to churn.
- Social media: Analyzing social media activity can reveal public perception and sentiment about your brand. Social media can highlight trends, customer expectations, and emerging issues that might not be evident from internal sources of data.
Techniques for data cleaning and pre-processing
Once data is collected, it must be cleaned and pre-processed to ensure its quality. This process involves removing errors, inconsistencies, and irrelevant information. Techniques such as normalization, handling missing values, and outlier detection are crucial steps in this phase.
Normalization standardizes data, making it comparable across different sources. Managing missing values ensures that gaps in data do not skew the model’s predictions, and outlier detection helps in identifying and managing anomalous data points that could distort the results.
High-quality pre-processing ensures that the data fed into the predictive model is accurate and reliable.
Ensuring data privacy and security
Data privacy is a significant concern that must be addressed when handling customer data. Businesses must comply with data protection regulations, such as the European Union's General Data Protection Regulation (GDPR), and implement robust security measures.
Protecting user data involves practices like anonymizing sensitive information and obtaining explicit consent for data usage. These measures not only comply with legal standards but also build trust with customers, reassuring them that their data is handled responsibly.
Comprehensive security measures, such as encryption and regular audits, help safeguard data against breaches and misuse.
💡 Takeaway: Start by defining your objectives, such as reducing attrition and understanding reasons for potential departures. Next, prepare comprehensive and accurate data from various sources and focus on key features influencing churn, such as demographic, support, usage, contextual, and behavioral data. Finally, build and refine your predictive model using machine learning techniques ensuring continuous monitoring and adaptation to changing customer behaviors.
How can Digi Storms help in reducing churn
Digi Storms helps SaaS companies reduce churn through tailored lifecycle email strategies. Our expert team uses data-driven insights to create personalized campaigns that engage and retain customers.
We focus on understanding customer behavior, effective segmentation, and delivering targeted content. Our advanced analytics track campaign performance, allowing continuous optimization.
Contact us now to build stronger customer relationships, increase satisfaction, and ensure long-term customer loyalty for your business.